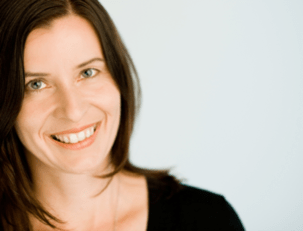
Birgit Gray
Innovation Manager
Broadcasters and media companies are implementing technologies powered by Artificial Intelligence (AI) and Machine Learning (ML) across the value chain. We see countless use cases for AI based automation or support and new opportunities keep emerging. So far, the focus has been on the usefulness of AI systems in terms of accuracy and performance in relation to a specific task. This is now changing with a wider uptake of AI, new capabilities for ML and public debates on this technology.
Birgit Gray from DW Innovation provides insight into Responsible AI and shares her activities in the AI4Media project related to making AI components in media tools more trustworthy.
Moving from AI to Responsible AI
Many media organisations now look at AI related risks, concerns of staff, acceptance levels and the degree of trust into outcomes or predictions. As in other industries, they might publish corporate AI guidelines or ethical principles for the use of AI in their organisation. Globally, there is dynamic development in the field of Responsible and Trustworthy AI, going beyond accuracy with dimensions such as Fairness, Privacy, Explainability, Robustness, Security, Transparency and Governance. In addition, specific AI regulation is emerging, for example the planned AI Act by the European Commission. While the principles of responsible AI seem to be well established, their implementation in AI systems and services remains complex and challenging.
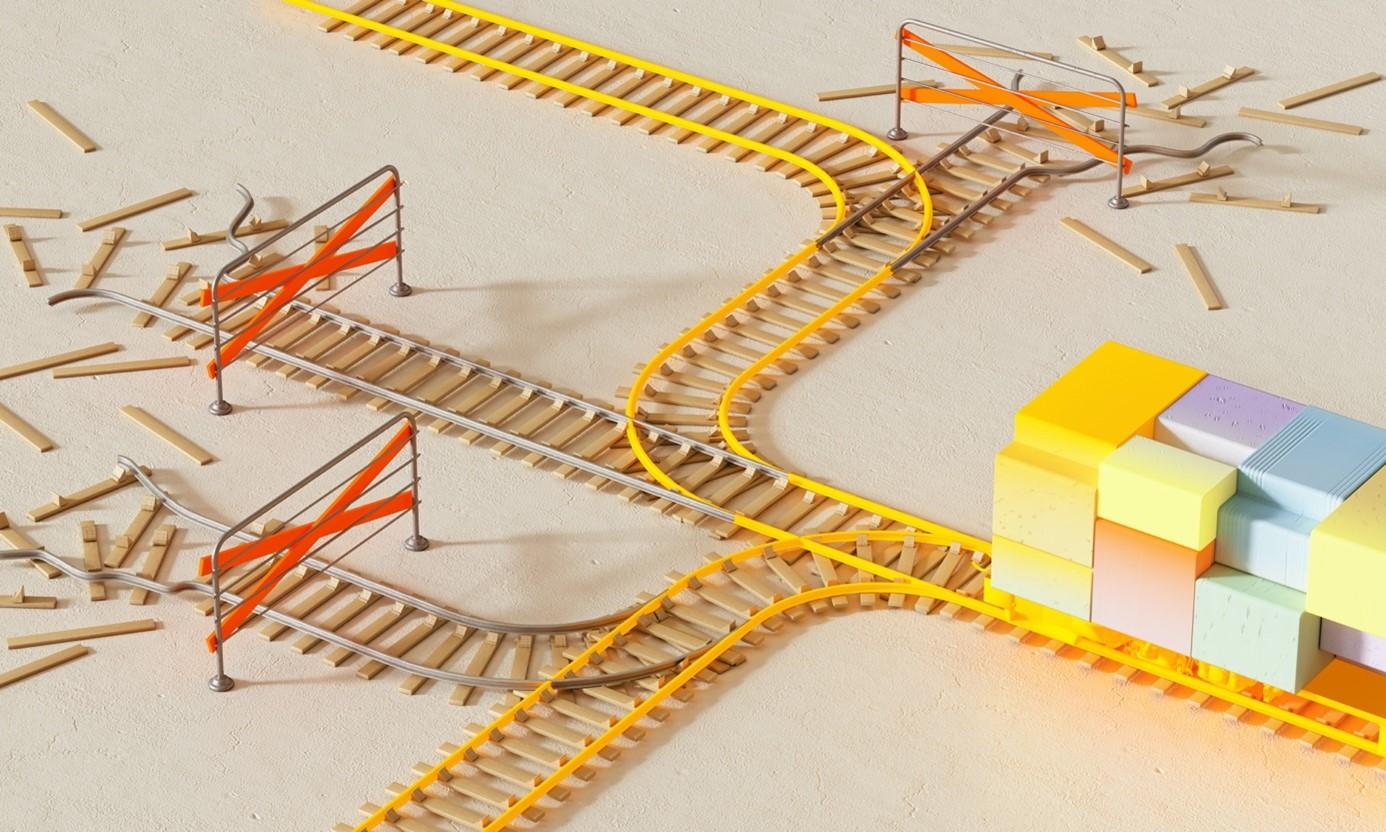
External AI Components
Media companies can be both: AI system developer and user of AI systems from third parties. When creating their own AI systems, they are in control of implementing aspects of responsible and trustworthy AI as required. This is different when deploying AI-powered media systems, content support tools or services that are provided and/or operated by external technology providers. AI related documentation may not be sufficient to judge if and to what extent the dimensions of trustworthy AI have been considered. This can leave AI related managers with a set of unanswered questions, for example, in relation to compliance with their corporate AI guidelines. Further, end users of AI driven support tools, such as journalists, might not easily trust the outcomes of an AI service or functionality.
Exploring Trustworthy AI Components in the AI4Media Project
With 30 European partners from the media industry, research institutes, academia, and a growing network of stakeholders, the EU co-funded AI4Media research project has several dimensions: it conducts advanced research into AI technologies related to the media industry, develops Trustworthy AI tools, integrates research outcomes in seven media related use cases, analyses the social and legal aspects of AI in Media, runs a funding programme for applied AI initiatives and establishes the AI Doctoral Academy AIDA.
Deutsche Welle (DW) runs one of the practical use cases in this project together with ATC iLab. Based on our requirements, we received a set of advanced AI functionalities from two of the project’s research partners: The MeVer Group at CERTH-ITI and Fraunhofer IDMT. These were integrated for testing and refinement into our Truly Media platform for content verification, which has been jointly developed by DW and ATC iLab and is operated by ATC.
These new AI components are designed to support users of the tool, journalists, and verification experts, with specific workflow tasks such as detecting deepfakes, synthetic audio, manipulation in photos or copy-move forgery in audio files. The AI services can help users in this complex, manual process by giving predictions or pointing to areas on which to focus human analysis. The final decision, whether a content item is synthetically generated or manipulated, remains with the human analyst.
For this reason, it is important that the end user of the media tool understands and trusts the outcome/prediction of an AI service that has been integrated into our tool. In addition, managers are interested in evaluating aspects of responsible AI, also in the context of organisational strategic AI guidelines (Governance). For one of the new AI services, the Deepfake Detection Service from CERTH-ITI, DW developed specific requirements for Trustworthy AI to address the above issues. Firstly, to obtain Transparency information for the AI component, e.g., related to the AI model, intended use or datasets. Secondly, to gain an understanding of the Robustness of the function in the event of any malicious adversarial attack performed on the machine learning model. Further, we needed information to what extent general responsible AI issues had been considered by the creators of the component.
How we Enhanced the Transparency of an AI Component
Transparency of an AI component can be achieved with the provision of a Model Card, a Fact Sheet or similar detailed documentation that is not always available for AI services. This gives descriptive transparency information and is not to be mixed up with the algorithmic improvement of the Explainability of an underlying Neural Network.
For the Deepfake Detection Service, DW opted for the Model Card approach, which was originally proposed in a paper by Margaret Mitchell and has since gained popularity in the AI community. It follows a structured approach to transparency information provision, including Model Details, Intended Use, Relevant Factors, Metrics, Evaluation/Training Data, Quantitative Analyses, Ethical Considerations and Caveats/Recommendations. Following the request from DW, the creator of the Deepfake Detection Service, CERTH-ITI, developed a two-page Model Card containing the above information. This document gives AI technology managers a much better understanding of the component developer, the intended purpose of the function, the scope and limitations, the types of datasets involved and its performance level. Such knowledge is relevant for a functional review as well as for assessing compliance with Responsible AI guidelines.
How we Evaluated the Robustness of an AI Component
The evaluation of Robustness relates to the overall resilience of an AI model against various forms of attacks. For example, an adversary could obtain access to the deployed model of the component and perform very minor imperceptible alterations to the input data to significantly reduce the accuracy of such a model.
While requested by DW as the user of the Deepfake Detection component, the algorithmic evaluation of its robustness was achieved through close cooperation between the component provider (CERTH-ITI) and the expert partner in AI4Media for algorithmic robustness technologies (IBM Research Europe - Dublin). Using the open source Adversarial Robustness Toolbox (ART), CERTH-ITI and IBM evaluated the performance of the Deepfake Detection service by deliberately applying adversarial attacks on the Deepfake Detection component’s AI model.
Resulting changes in the performance level of the ML model have been made transparent with a description in the Model Card. This enables comparison to the original performance evaluation results, which were also provided in the Model Card. From a business point of view, this approach allows for an assessment of how secure (and reliable) this AI model is in the context of possible attacks.
Accessible Transparency Information for Different Target Groups
An evaluation of the Model Card revealed that the technical language and AI jargon used are not easily understood by all target groups in a media organisation that require this information. Consequently, DW developed a business-oriented user guide that follows a Q&A format and uses non-technical language. The development of this document required further explanation and input from the AI component provider to achieve a version of the Model Card that is “understandable” by managerial target groups. In cooperation, DW and CERTH-ITI also added further information related to legal, privacy, fairness, explainability, security and sustainability aspects, as well as other social/ethical issues. The aim was to raise awareness for these issues in the context of this functionality and to point out open issues that have recently emerged where the research community is still exploring solutions.
Based on this user guide, DW plans to explore further tailored versions, also for end users of the Truly Media tool for integration into the Truly Media user interface.
Lessons Learned
While responsible AI principles and guidelines are now commonplace, the provision of trustworthy AI systems is at the beginning. Our exploration of trustworthy AI in media tools has shown that giving information about the AI related managerial and user context in a media organisation to AI technology providers is a good starting point. Generally, it may be easier to commence with descriptive transparency information and then move towards more complex algorithmic trustworthy AI improvement if and where required for a given use case (e.g., Fairness, Privacy, Robustness or Explainability). Although instruments like the Model Card provide an essential basis, further effort needs to be made for making trustworthy AI information accessible to different target groups as well as end users of an AI driven tool.
With a view to scaling our explorative work, several questions remain: Which party should provide what kind of responsible AI input, how to organise the process of cooperation between users in a media organisation and external AI component providers, and what is the role of upcoming regulation and certification approaches?
For resources from the AI4Media project, visit the results section on the project’s website, containing White Papers from the use cases, an in-depth report on AI & Media, as well as specific reports on legal, social, and trustworthy AI aspects. The project also provides open data sets and AI components via Europe’s AI-On-Demand Platform.